Extant hyperparameter optimisation approaches that search out optima within large combinatorial search spaces randomly or non-specifically, when viewed through the ‘No-Free-Lunches’ theorem, are a priori unjustifiable. An iterative Robust Search (iRoSe) hyperparameter optimisation framework is formulated, that robustifies optimisation in a coevolutionary, adaptive manner, by leveraging gain in Signal-to-Noise Ratio (SNR). iRoSe abstracts out complexity of the learning algorithm through ‘macro-modelling’ in terms of signal, control, noise and response. Designed sets of ‘proportionally balanced’ trials are run, followed by a ‘main effects analysis’ of SNR from hyperparameter values. iRoSe iteratively converges onto configurations that produce effective gain in performance characteristic.
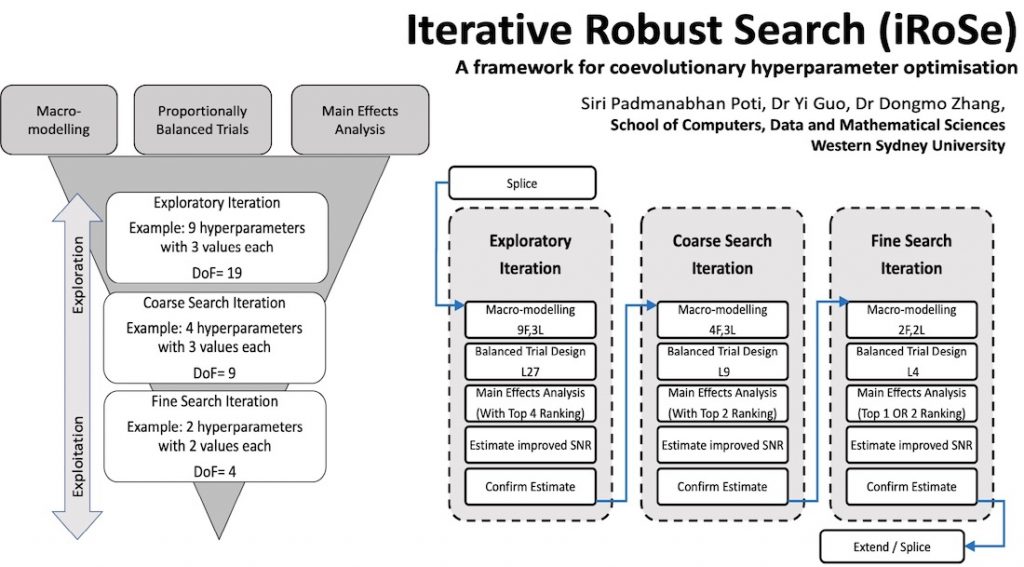
HDR Student: Siri Padmanabhan Poti
Supervisory Panel: Dr Yi Guo and Asso. Prof. Dongmo Zhang